CPG supply chains are facing challenging times due to consumer behavior changes, demand volatility, and more. Tredence offers CPG analytics services to leverage the power of CPG data insights for inventory optimization and supply chain automation.
A perfectly stocked shelf with a careful mix of products/SKUs is something that retailers love to see. But it takes much more than wishful thinking to master this inventory situation. What can CPGs do to hone their efforts of precision stocking? It requires a combination of predictive analytics and customer insights.
AI-backed predictive analytics provides CPG companies with the power to peer into the future and forecast shifts in demand. But that’s also insufficient. It’s essential to have an in-depth knowledge of the consumer. Each consumer interaction, comment, and preference forms a deeper, finer-grained profile. With proper analytics and predictive capabilities, CPGs can stock the right product at the right moment.
According to a 2019 BCG study, CPG firms who used data-driven personalization achieved sales increases of 6–10%, two–three times quicker than other firms in their industry.
There’s another important challenge that CPGs are struggling with. Recent disruptive events, inflation, rising costs, and scarcity of raw materials have put a lot of pressure on supply chains.
In addition, consumer behaviors are changing too and shaping the market dynamics like never before. Consumers now expect frictionless experiences, instant gratification, and a high level of personalization.
In the actual scenario and considering all major factors, how can companies rethink their strategies to keep up with these changes?
The answer lies in harnessing the transformative power of CPG data insight and digitalization.
According to a 2022 study, digitalization and AI in the supply chain have positively affected a company’s performance.
CPG data insights can prove extremely valuable for supply chain management and inventory optimization. Unlocking the power of data opens the door to a more holistic and clear vision of the supply chain in all its steps, understanding where pitfalls are and quickly intervening to fix setbacks. Moreover, it allows companies to gain customer insights to better understand demand, needs, and preferences.
So, how can we utilize CPG data insights for inventory optimization and better supply chain management?
Data collection: How to get CPG data insights
In CPG, every transaction is a gold mine of data. From purchase and shopping behavior to consumer trends – any insights at any stage of the consumers’ buying journey is pivotal in the inventory planning process. In fact, according to Gartner, by 2025, 70% of enterprises will move their focus from big to small and wide data, providing more context for analytics and making AI less data-hungry. But how do we translate the vast ocean of data into profitable outcomes?
It all start with data collection. But with a staggering 2.5 quintillion bytes of data generated each day, the first step of gathering the valuable ones is a challenge in itself. Advanced CPG data analytics platforms use AI to collect relevant CPG data from all sources and then employ machine learning-enabled Ops processes, like MLOps, to reduce noise and clear out non-relevant data.
Important data sources are:
- Sales records
- Point-of-sale systems
- Customer feedback
- Market research
- Social media monitoring
Once collected, data must be analyzed to identify patterns, trends, and demand fluctuations. Data analytics in the CPG industry unlocks customer insights that help companies understand seasonality, trends, and their impact on product demand. Businesses need to be aware of the impact of factors like holidays, weather, promotional periods, and product lifestyle changes; the analysis of historical data plays a relevant role in scenario planning.
AI-powered simulations for accurate forecasting
In the uncertain times we’re living in, scenario planning is gaining more and more relevance. Stepping up your prediction and analysis capabilities by leveraging CPG data insights means gaining a competitive advantage.
Brands have historically employed econometrics and statistical forecasting methods to predict future market demand and organize inventory. However, these methods present important limits, as they cannot simultaneously model different types of variations. So, is there a solution? Yes, and it’s machine learning.
Machine learning can drive predictive analysis beyond just estimating demand. Combining historical data with real-time inputs generates market and customer insights under different conditions and makes data-driven predictions.
Which factors must be considered for accurate predictions about CPG demand and future sales?
- Historical data
- Price and promotion strategy
- Marketing campaigns
- Economic indicators (consumer spending, inflation, etc.)
- External events
Analyzing consumer data helps your company understand what will happen to consumer demand under different circumstances, like a new competitor entering the market or changes in consumers’ spending power due to inflation. Running several scenarios allows us to prepare for various possibilities, prioritizing inventory optimization.
Automation in the supply chain: Avoid understocking and overstocking
According to a study from IHL Group, overstocking and understocking cost retailers 1.1$ trillion in lost revenue, with the former responsible for 3.2% of revenue loss and the latter for 4.1%.
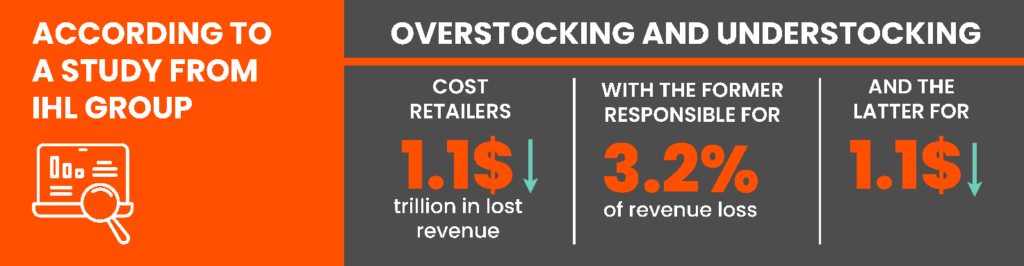
Every business serious about its revenue growth management can’t ignore these numbers and the issue they show. Developing inventory policies that align with CPG data insights allows for determining optimal reorder points and safety stock levels, enabling inventory optimization and a stronger, more resilient supply chain.
CPG data analytics and technology also enable automation: an automated, AI-powered dynamic system that adjusts inventory levels based on real-time data, such as current stock, lead times, and demand fluctuations. In this model, data analysis, forecasting, and replenishment are automated based on CPG data insights, enabling more efficient and accurate inventory optimization. This dynamic system is a state-of-the-art solution to understocking and overstocking because it aligns inventory levels with demand patterns and real-time data.
Monitoring supply chain performance for inventory optimization
Once put in place, this automated, data-driven supply chain model must be constantly monitored to ensure maximum efficiency. Each day your supply chain generates more data, from customer insights to sales levels, and you have to process this data and incorporate it into your CPG data analysis.
AI-powered systems continuously learn from consumers data and refine logistics and inventory management strategies accordingly. They reassess and adjust supply chain strategies based on evolving market dynamics, customer insights and preferences, changes in demand, and emerging trends.
Incorporating AI and machine learning into the supply chain is not without problems, as it adds a layer of complexity that most companies are not able to manage alone. CPG data companies like Tredence help businesses on their path to automation and digitalization, offering CPG analytics solutions for revenue growth management.
Leveraging CPG data insights and customer insights
CPG supply chains face an important challenge: navigate uncertain times and find on-shelf availability solutions to avoid understocking, overstocking, and the associated losses.
Gartner takes a strong stand regarding the future of inventory optimization and CPG Data Analytics:
“Given today’s volatile and disruptive environment, supply chain organizations must become more flexible, and the solution is digitalization.”
And it predicts that by 2026, 75% of large enterprises will have adopted AI solutions and smart robots in their warehouse operations.
CPG data insights allow the development of a new supply chain model with increased efficiency, resilience, and responsiveness for inventory optimization. Advanced customer insights and demand forecasting nurture this dynamic system, reducing losses and improving revenue growth management.